Highlights
-
Developed a 21-language, fast and high-fidelity neural text-to-speech technology
-
The developed model can synthesize one second of speech at high speed in only 0.1 seconds using a single CPU core, which is about eight times faster than the conventional methods
-
The developed model can realize fast synthesis with a latency of 0.5 seconds on a smartphone without network connection
-
The technology is expected to be introduced into speech applications, such as multilingual speech translation and car navigation
The Universal Communication Research Institute of the National Institute of Information and Communications Technology (NICT, President: TOKUDA Hideyuki, Ph.D.) has successfully developed a 21-language, fast and high-fidelity neural text-to-speech technology. The development of this technology has made it possible to synthesize one second of speech at high speed in just 0.1 seconds using a single CPU core, which is about eight times faster than the conventional methods. This technology also enables fast synthesis with a latency of 0.5 seconds on a mid-range smartphone without network connection (see Figure 1).
Furthermore, the developed 21-language neural text-to-speech models are installed on the server of VoiceTra, a multilingual speech translation application for smartphones operated by NICT, and have been made available to the public. In the future, the technology is expected to be introduced into various speech applications, such as multilingual speech translation and car navigation through commercial licensing.
These results will be presented at INTERSPEECH 2024 Show & Tell, an international conference hosted by the International Speech Communication Association (ISCA) in September 2024.
Background
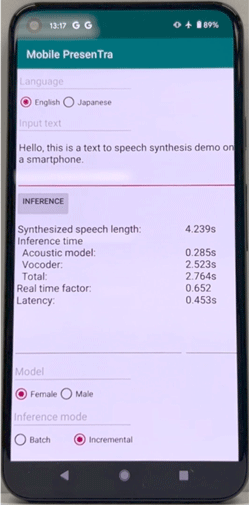
Figure 1: Text-to-speech model implemented on a mid-range smartphone
(Movie: https://youtu.be/VckvQvhlcSs)
(Movie: https://youtu.be/VckvQvhlcSs)
The Universal Communication Research Institute, NICT is conducting R&D of multilingual speech translation technology to realize spoken language communication that transcends language barriers. The outcomes of R&D have been released to the public as a field experiment on VoiceTra, a speech translation application for smartphones, and many other implementations have been made in society through commercial licensing. Text-to-speech technology, which can synthesize the translated text as human speech, is very important for the realization of multilingual speech translation technology, as well as automatic speech recognition and machine translation. The synthesized sound quality of text-to-speech has improved dramatically in recent years thanks to the introduction of neural network technology, and it has reached a level comparable to that of natural speech, however, the huge amount of calculation was a major issue; thus impossible to synthesize on a smartphone without network connection.
Furthermore, NICT is currently conducting R&D on multilingual simultaneous interpretation technology. In simultaneous interpretation, it is required to output the translated speech one after another without waiting for the speaker to finish speaking. Therefore, it is indispensable to further accelerate text-to-speech as in automatic speech recognition and machine translation.
Achievements
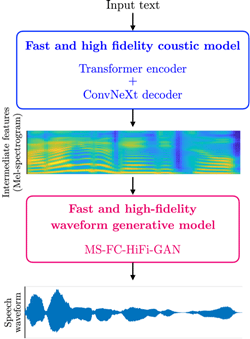
Figure 2: Fast and high-fidelity neural text-to-speech model architecture
Text-to-speech models are typically constructed from an acoustic model that converts input text into intermediate features, and a waveform generative model that converts intermediate features into speech waveforms.
While neural networks (Transformer encoder + Transformer decoder), which are widely used in machine translation, automatic speech recognition, and large language models (e.g. ChatGPT) are the mainstream in acoustic modeling for neural text-to-speech, we have introduced high-speed, high-performance neural networks (ConvNeXt encoder + ConvNeXt decoder), which have been recently proposed in image identification, into the acoustic model, and achieved three times faster synthesis without performance degradation compared with the conventional methods.
In 2021, we introduced MS-HiFi-GAN, in which the signal processing method [2-4] is represented with a training-capable neural network, by extending the conventional model, HiFi-GAN, which can synthesize speech equivalent to human speech, and achieved two times faster synthesis without synthesis performance degradation [5]. In 2023, we successfully developed MS-FC-HiFi-GAN by further accelerating the MS-HiFi-GAN, and achieved four times faster synthesis without synthesis performance degradation compared with the conventional HiFi-GAN.
As the culmination of these achievements, we have developed a novel, fast and high-quality neural text-to-speech model using an acoustic model (Transformer encoder + ConvNeXt decoder) and a waveform generation model (MS-FC-HiFi-GAN) as shown in Figure 2. As a result, the developed model is capable of synthesizing one second of speech at high speed in only 0.1 seconds using a single CPU core, which is about eight times faster than the conventional models. In addition, by introducing a method where incremental synthesis is only applied to the waveform generative model (see Figure 3), the developed model achieved fast synthesis with a latency of 0.5 seconds on a mid-range smartphone without network connection nor synthesis performance degradation. This eliminates the need for internet connection or conventional server-based synthesis and enables high-quality neural text-to-speech on smartphones, PCs, and other devices with reduced communication costs. Furthermore, incremental synthesis processing also makes it possible to immediately synthesize translated text in multilingual simultaneous interpretation.
Since March 2024, the developed technology has been used for neural text-to-speech in 21† of the languages supported in VoiceTra and has been made available to the public.
†21 languages: Japanese, English, Chinese, Korean, Thai, French, Indonesian, Vietnamese, Spanish, Myanmar, Filipino, Brazilian Portuguese, Khmer, Nepali, Mongolian, Arabic, Italian, Ukrainian, German, Hindi, and Russian
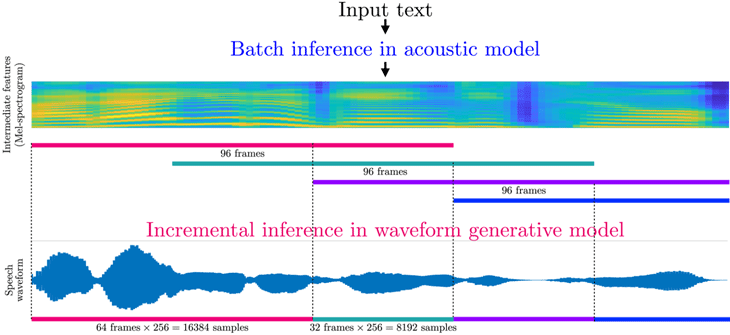
Figure 3: Low-latency synthesis can be realized by incremental inference only applied to waveform generative model.
Future prospects
In the future, we will promote social implementation, specifically for smartphone applications, etc. such as multilingual speech translation and car navigation systems through commercial licensing.
Article information
Journal: Proceedings of INTERSPEECH 2024
Title: Mobile PresenTra: NICT fast neural text-to-speech system on smartphones with incremental inference of MS-FC-HiFi-GAN for low-latency synthesis
Authors: Takuma Okamoto, Yamato Ohtani, Hisashi Kawai
References
[1] T. Okamoto, Y. Ohtani, T. Toda and H. Kawai, "ConvNeXt-TTS and ConvNeXt-VC: ConvNeXt-based fast end-to-end sequence-to-sequence text-to-speech and voice conversion," in Proc. ICASSP, Apr. 2024, pp. 12456-12460.
[2] T. Okamoto, K. Tachibana, T. Toda, Y. Shiga and H. Kawai, "Subband WaveNet with overlapped single-sideband filterbanks," in Proc. ASRU, Dec. 2017, pp. 698-704.
[3] T. Okamoto, K. Tachibana, T. Toda, Y. Shiga and H. Kawai, "An investigation of subband WaveNet vocoder covering entire audible frequency range with limited acoustic features," in Proc. ICASSP, Apr. 2018, pp. 5654-5658.
[4] T. Okamoto, T. Toda, Y. Shiga and H. Kawai, "Improving FFTNet vocoder with noise shaping and subband approaches," in Proc. SLT, Dec. 2018, pp. 304-311.
[5] T. Okamoto, T. Toda and H. Kawai, "Multi-stream HiFi-GAN with data-driven waveform decomposition," in Proc. ASRU, Dec. 2021, pp. 610-617.
[6] T. Okamoto, H. Yamashita, Y. Ohtani, T. Toda and H. Kawai, "WaveNeXt: ConvNeXt-based fast neural vocoder without iSTFT layer," in Proc. ASRU, Dec. 2023.
[7] H. Yamashita, T. Okamoto, R. Takashima, Y. Ohtani, T. Takiguchi, T. Toda and H. Kawai, "Fast neural speech waveform generative models with fully-connected layer-based upsampling," IEEE Access, vol. 12, pp. 31409-31421, 2024.
About “VoiceTra”

<How to use>
- Support webpage: https://voicetra.nict.go.jp/en/
- Video on how to use: https://voicetra.nict.go.jp/en/picture.html
VoiceTra is a registered trademark of the National Institute of Information and Communications Technology.