Highlights
-
Humans take more risks when interacting with facial expressions shown on avatars rather than real human faces.
-
This shift in risk-taking behavior is linked to activity in the amygdala.
-
The findings offer new insights into both the advantages and the cautionary aspects of communication via avatars.
Abstract
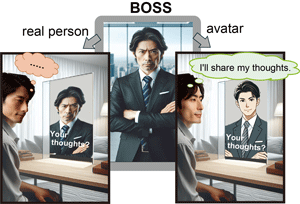
Figure 1 Example scenario
How expressing opinions in an online meeting may feel different depending on whether a supervisor’s face is shown directly or represented as an avatar.
A research team led by Dr. TANAKA Toshiko and Dr. HARUNO Masahiko at the National Institute of Information and Communications Technology (NICT, President: TOKUDA Hideyuki Ph.D.), investigated how avatar-mediated communication affects human decision-making. They discovered that participants were more likely to take risks when facial expressions (such as admiration or contempt) were displayed by avatars than when the same expressions were shown on real human faces. This increase in risk-taking was found to result from a more favorable valuation of the "uncertainty" of facial feedback in the avatar condition. Furthermore, fMRI analysis revealed that this valuation of uncertainty depends on activity in the amygdala.
These results offer important insights into how avatar-based social communication, such as in virtual and augmented reality, can affect human decision-making, and highlight the amygdala's critical role in this process. The findings were published in the April 22, 2025 issue of the journal PLOS Biology, which features high-impact research in the life sciences.
Background
In recent years, avatar-mediated communication has rapidly spread in contexts such as online meetings and customer service. However, scientific studies on how avatars influence human cognition and decision-making are still in their early stages. In particular, little is known about how behavior changes when a communication partner's facial expressions are shown via avatars rather than real faces. For example, situations such as expressing opinions during online meetings or shopping in virtual stores increasingly involve avatars as communication partners. Understanding how avatars' facial expressions influence risk-related decisions in such settings is crucial.
Achievements
In this study, participants performed a risk decision-making task while undergoing fMRI scanning. In each trial, they were presented with two options and asked to choose their preferred one:
- A "safe" option: a guaranteed small reward (e.g., 80 yen with 100% probability)
- A "risky" option: a larger reward with lower probability (e.g., 300 yen with 33% probability)
Before the task, participants had a face-to-face meeting with a same-gender observer. During the task, when participants selected the risky option:
- Success was followed by the observer showing an admiring facial expression
- Failure was followed by a contemptuous expression
These expressions were shown either using the observer's real face (Human condition) or an avatar face (Avatar condition), switching every 6 to 13 trials (see Figure 2, left).
Results showed that participants made more risky choices in the Avatar condition than in the Human condition, particularly when the risky option had only a slightly higher expected value (see Figure 2, right).
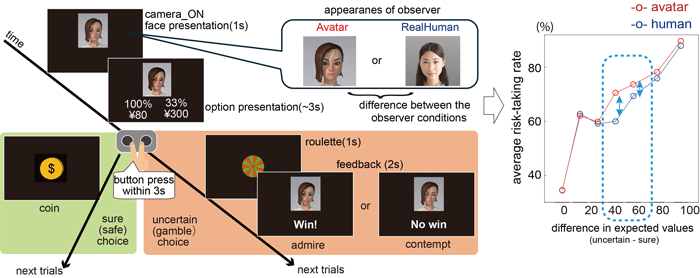
Figure 2. (Left) Risk decision-making task. Participants chose between a "safe" and "risky" option. While making choices, they were observed via camera by an on-screen face—either a real human or an avatar. If they selected the risky option, the outcome (win or no win) was followed by the observer displaying a facial expression: admiration for wins, contempt for losses. The avatar and human face conditions alternated every 6 to 13 trials. When the safe option was selected, the participant received the reward and moved on to the next trial. Reward values and probabilities changed each trial, with one option always being 100%.(Right) Increase in risk-taking under the avatar condition. The red line shows the average risk-taking rate under the Avatar condition, and the blue line under the Human condition. The x-axis represents the expected value difference between the two options. When the risky option was only slightly better (moderate expected value differences), participants were more likely to choose it under the Avatar condition.
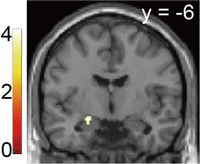
Figure 3 Amygdala activity reflecting valuation of feedback uncertainty. The amygdala encoded individual differences in how uncertain facial feedback was interpreted, explaining variations in risk-taking behavior across participants.
The increase in risky decision-making was explained by a more favorable valuation of the uncertainty of which facial expression would be shown. This valuation was more positive in the Avatar condition.
fMRI analysis further revealed that this valuation of uncertainty was associated with activity in the amygdala (see Figure 3).
Future Prospects
This study demonstrates that avatar-mediated communication influences human risk-taking behavior and that this effect is linked to the amygdala. The researchers plan to further investigate how factors such as the avatar's gender or age, individual personality traits, and other types of decision-making tasks are influenced by avatars.
They also aim to explore how avatars can be effectively used to support decision-making in real-world settings such as education and interpersonal support, while also identifying potential risks associated with avatar use.
Article information
Authors: Toshiko Tanaka, Masahiko Haruno
Title: Feedback from an avatar facilitates risk-taking by modulating the amygdala response to feedback uncertainty
Journal: PLOS Biology
DOI: 10.1371/journal.pbio.3003122
This research was supported in part by the following programs: JST Moonshot R&D Program “Realization of a society where people are free from limitations of body, brain, space, and time by 2050,” project: “Realizing a society where avatars coexist and empower all individuals”, JST CREST Program “Exploration and application of multi-sensing systems in biological environments,” project: “Decoding multi-world predictive coding in cyber society” (Principal Investigator: HARUNO Masahiko) and Grant-in-Aid for Transformative Research Areas (A) “Decoding and manipulating brain dynamics that induce early behavioral changes, opening up multi-layered biology.”
All experiments in this study were approved by the NICT ethics committee. Informed consent was obtained from all participants after explaining the procedures of the study.
Illustrations in the background section were adapted from images generated using Microsoft Copilot.