National Institute of Information and Communications Technology
Home > Press Release > Ensemble Tractography
A method to improve the identification of white matter pathways in living human brain
February 12, 2016
Abstract
Hiromasa Takemura, a researcher in Center for Information and Neural Networks (CiNet), NICT has proposed a new method named “Ensemble Tractography”, which improves the performance to identify white matter pathways from living human brains. This method aims to combine the advantage of multiple tractography methods in order to overcome the limitation arises from selecting a single tractography algorithm and parameter settings. This method will contribute to advance future studies to understand the nature of massive information transmission throughout white matter pathways in the human brain.
Achievements
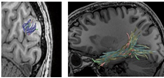
Ensemble Tractography
The human brain comprises a number of white matter pathways involved with the communication between distant brain regions. Recent advances in diffusion magnetic resonance imaging (diffusion MRI) and tractography enable us to identify white matter pathways from the living human brain. This progress opens a new avenue for studying human white matter in relation to disease, development and behaviors.
Tractography estimates the trajectory of white matter pathways by tracking the orientation of diffusion MRI signal in each data point. Nowadays, a variety of tractography algorithms and softwares are widely used, each of which estimates white matter pathways based on different computational principles. In any algorithm, users are asked to select parameter values for performing the tractography. As a common practice, users chose a specific algorithm and a parameter set in order to analyze the diffusion MRI data. Choosing an algorithm and parameter settings poses a great challenge, as the results will significantly depend on that choice.
The top and middle panels of the figure below illustrate this problem. The panels show the estimates of white matter pathways using the same dataset with different parameters in tractography. In the top panel, a short-range pathway (U-fibers) is clearly identified whereas a long-range pathway (Inferior Longitudinal Fasciculus; ILF) is not well supported. In the middle panel, the ILF is clearly supported whereas the U-fiber is not. Thus, even when we analyze the same dataset, the estimates of the white matter pathways significantly depend on how we chose the parameters. Researchers have noted that this dependency makes it difficult for interpreting tractography results in a neurobiological context.
In this research, Hiromasa Takemura and collaborators (Franco Pestilli, Indiana Univ.; Cesar Caiafa, CONICET; Brian Wandell, Stanford Univ.) proposed a new method named “Ensemble Tractography”. In this method, white matter pathways are estimated using multiple algorithms and parameter settings, and the results are combined to create a super-set candidate model of white matter pathways. Next, Linear Fascicle Evaluation (LiFE; Pestilli et al., 2014, Nat Methods) is applied to select the valid pathways from the candidate model. Essentially, Ensemble Tractography enables us to take advantages from multiple methods; a super-set candidate is created, and LiFE selects the valid estimates from candidate.
The bottom panel in the figure describes the white matter pathways identified by Ensemble Tractography. Ensemble Tractography identified both U-fiber and the ILF, whereas conventional tractography with single parameter setting is biased to one or the other (top and middle panels). Moreover, Hiromasa and colleagues also showed that Ensemble Tractography provides higher model accuracy of white matter connections as compared with previous methods.
This paper appeared in the journal PLOS Computational Biology on February 4, 2016.
Future perspectives
Ensemble Tractography addresses a widely recognized problem with tractography methods, namely, that the results depend significantly on the choice of algorithms and parameters. This advancement will improve the quality and reliability of tractography results, providing further opportunities for a more complete understanding about how information is transmitted in the human brain.
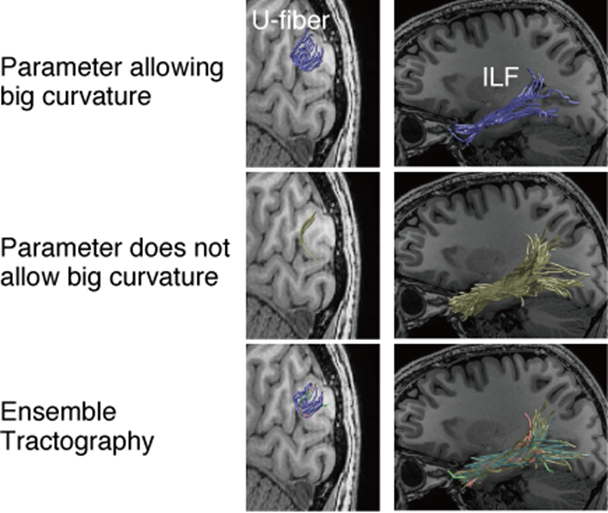
Figure. White matter pathways identified by conventional tractography and ensemble tractography.
The two columns compare short-range pathway (left, U-fiber) and long-range pathway (the ILF) identified using the conventional and ensemble tractography. In conventional tractography methods, users must set a key parameter, curvature threshold, for identifying white matter pathways from diffusion MRI data. As a result, estimated white matter pathways are extremely different depending on the parameter selection (top panel, parameter allowing big curvature; middle panel, parameter does not allow big curvature). Top panel is example results using parameter allowing big curvature, and show a dense set of U-fiber but a thin set of ILF. Conversely, middle panel is example results using parameter which does not allow big curvature, which shows sparse U-fiber and dense ILF. Ensemble Tractography (bottom panel) takes the advantages of both methods, and supports both U-fiber and ILF. Figure is reproduced from Takemura et al. (2016) PLOS Computational Biology.
“Ensemble Tractography”
Hiromasa Takemura, Cesar F. Caiafa, Brian A. Wandell, Franco Pestilli (2016) PLOS Computational Biology, 12(2): e1004692. doi:10.1371/journal.pcbi.1004692
Hiromasa Takemura, Cesar F. Caiafa, Brian A. Wandell, Franco Pestilli (2016) PLOS Computational Biology, 12(2): e1004692. doi:10.1371/journal.pcbi.1004692
Technical Contact
Hiromasa Takemura
Brain Networks and Communication Laboratory
Center for Information and Neural Networks (CiNet)
Tel: +81-080-9098-3285
E-mail:
Media Contact
Sachiko Hirota
Public Relations Department
Tel: +81-42-327-6923
Fax: +81-42-327-7587
E-mail: